Introduction
Please see the background information about Twitter API v2 in my last blogpost Twitter API: Door To Social Media Analysis I. In this blogpost, I will replicate the Tweets extraction porcess of Bartov, Faurel, and Mohanram (2018, TAR), which found that opinions of individuals tweeted just prior to a firm’s earnings announcement can predict its earnings and announcement returns.
From a perspective of implementation, we need to get all the tweets which mentioned a given firm in a specified time range. Consistent to the paper’s definition, the targeted tweets should:
- Include stock symbols preceded by the dollar sign (e.g., $AAPL for Apple Inc.; $PEP for PepsiCo Inc.)
- In which the stock symbols belong to Russell 3000 firms
- Be released between March 21, 2006 and December 31, 2012
RoadMap
-
Import access token from the environment variables and put the token into the headers. Regarding how to get access token and make it an environment variable, please check Get Access to Twitter API.
-
Set parameters for queries. Regarding the available fields and their usages for Tweet object, please check Fields of Tweet Object.
By specifying
start_time
,end_time
, andquery
, I require the returned tweets:- Is released no earlier than
start_time
and no later thanend_time
- Contain the ticker of a firm
firm
preceded by the dollar sign
By specifying
tweet.fields
, I expect the following information for each tweet is returned:created_at
,public_metrics
,author_id
,lang
By specifying
max_results
, one can get 10 tweets for each request. This figure could be changed.1 2 3 4 5 6
# Input filter parameters: firm tickers, start time stamp, end time stamp # Optional params: start_time,end_time,since_id,until_id,max_results,next_token, # expansions,tweet.fields,media.fields,poll.fields,place.fields,user.fields def setpara(firm,start_time,end_time): query_params = {'start_time': "%s"%start_time, 'end_time':"%s"%end_time, 'query': "$%s"%firm, 'tweet.fields':"created_at,public_metrics,author_id,lang",'max_results': 10} return(query_params)
- Is released no earlier than
-
Submit a query and check the returned data
For now, we have obtained the necessary elements for submitting a query:
- Access token
bearer_token
- Query parameters
start_time
,end_time
, andquery
- Returned fields
created_at
,public_metrics
,author_id
,lang
- Parameter for page splitting
max_results
With those elements, we can submit our very first query and analyse the returned data to decide on how to clean it.
1 2 3 4 5 6 7 8 9 10 11 12 13 14 15 16 17 18 19 20 21 22 23 24 25 26 27 28 29 30 31
import requests, os, json # To set your environment variables in your terminal run the following line: # export 'BEARER_TOKEN'='<your_bearer_token>' bearer_token = os.environ.get("BEARER_TOKEN") search_url = "https://api.twitter.com/2/tweets/search/all" # Optional params: start_time,end_time,since_id,until_id,max_results,next_token, # expansions,tweet.fields,media.fields,poll.fields,place.fields,user.fields def setpara(firm,start_time,end_time): query_params = {'start_time': "%s"%start_time, 'end_time':"%s"%end_time, 'query': "$%s"%firm, 'tweet.fields':"created_at,public_metrics,author_id,lang",'max_results': 10} return(query_params) def create_headers(bearer_token): headers = {"Authorization": "Bearer {}".format(bearer_token)} return headers def connect_to_endpoint(url, firm, start_time, end_time): response = requests.request("GET", search_url, headers = create_headers(bearer_token), params=setpara(firm,start_time,end_time)) print(response.status_code) if response.status_code != 200: raise Exception(response.status_code, response.text) return response.json() def search(firm, start_time, end_time): json_response = connect_to_endpoint(search_url, firm, start_time, end_time) print(json.dumps(json_response, indent=4, sort_keys=True)) if __name__ == "__main__": search("AAPL", '2012-03-21T00:00:00Z', '2012-03-21T00:20:00Z')
If the code is executed successfully, we will get response displayed as follows (To save the space, I removed the 2nd to 9th tweets returned).
1 2 3 4 5 6 7 8 9 10 11 12 13 14 15 16 17 18 19 20 21 22 23 24 25 26 27 28 29 30 31 32 33 34 35 36 37 38
200 { "data": [ { "author_id": "215869072", "created_at": "2012-03-21T00:18:35.000Z", "id": "182259875408121856", "lang": "en", "public_metrics": { "like_count": 0, "quote_count": 0, "reply_count": 0, "retweet_count": 1 }, "text": "@FortuneMagazine Can #Apple's stock reach $1,368? http://t.co/wJlWogk1 $AAPL @albertosrt" }, ... { "author_id": "341817759", "created_at": "2012-03-21T00:06:37.000Z", "id": "182256865110003712", "lang": "en", "public_metrics": { "like_count": 0, "quote_count": 0, "reply_count": 0, "retweet_count": 0 }, "text": "was forced to buy a small position in $aapl today to offset shorts in other names, in at 594 with $3 trailing" } ], "meta": { "newest_id": "182259875408121856", "next_token": "1jzu9lk96gu5npvzel7a7nb3ogobtgzn9360fuunqj5p", "oldest_id": "182256865110003712", "result_count": 10 } }
- Access token
-
Update query paramters with
next_token
to get the returned data of the next pageClearly, the tweets are returned in a json format. Different from the fields we have requested (
created_at
,public_metrics
,author_id
,lang
), themeta
part is the key for jumping to the next page. If the meta has the elementnext_token
, that means there are more pages contained in your submitted query and we need to add the value ofnext_token
into the query parameters to get the returned data in the next page. Apparently, we need to repeat this procedure until the value ofnext_token
is empty.1 2 3 4 5 6 7 8 9 10 11 12 13 14 15 16 17 18
# Add the 'next_token' returned in the search result into the parameters for the subsequent query for the next page def updatepara(oldpara, next_token): newpara = {**oldpara,**{'next_token':next_token}} return(newpara) # Request the content of next page with updated parameters def dealjson(firm, start_time, end_time, json_response): headers = create_headers(bearer_token) # Retrieve the 'next_token' from the returned json next_token = csvjson(firm, start_time, end_time, json_response) while next_token: # If the 'next_token' is not empty, add it to the search parameters and submit a new query newpara = updatepara(setpara(firm,start_time,end_time), next_token) time.sleep(3) json_response = connect_to_endpoint(search_url, headers, newpara) next_token = csvjson(firm, start_time, end_time, json_response)
-
Record the request history and transfer the received data to csv format.
1 2 3 4 5 6 7 8 9 10 11 12 13 14 15 16 17 18 19 20 21 22 23 24
# Clean data received and export in csv format def csvjson(firm, start_time, end_time, json_response): # Record meta data of request history meta = json_response['meta'] callnum = meta['result_count'] try: next_token = meta['next_token'] except: next_token = False empty = (callnum==0) with open("/xx/meta.csv",'a') as g: now = time.strftime('%Y-%m-%d %H:%M:%S',time.localtime(time.time())) g.write(",".join([firm, start_time, end_time,now, repr(callnum), str(next_token)])+'\n') # Clean received json-formatted tweets and export in csv format if not empty: with open("/xx/%s.csv"%firm,'a') as f: w = csv.writer(f) tweets = json_response['data'] for tw in tweets: list = [tw['created_at'], tw['id'], tw['text'],tw['public_metrics']['retweet_count'],tw['public_metrics']['reply_count'],tw['public_metrics']['like_count'],tw['public_metrics']['retweet_count'],tw['author_id'], tw['lang']] w.writerow(list) return(next_token)
-
Iterate the above steps through the pre-specified ticker list.
1 2 3 4 5 6 7 8 9 10 11 12
# Input the ticker list tickerlist = open("/xx/code/tickerlist.txt",'r') def mainparse(firm,start_time,end_time): headers = create_headers(bearer_token) json_response = connect_to_endpoint(search_url, headers, setpara(firm,start_time,end_time)) dealjson(firm, start_time, end_time, json_response) if __name__ == "__main__": for ticker in tqdm(tickerlist): mainparse('$%s'%ticker.strip(),'2006-03-21T00:00:00Z','2012-12-31T23:59:59Z') time.sleep(1)
Sample Results
All th extracted tweets for a given firm are saved in a same csv file named by the firm’s ticker.
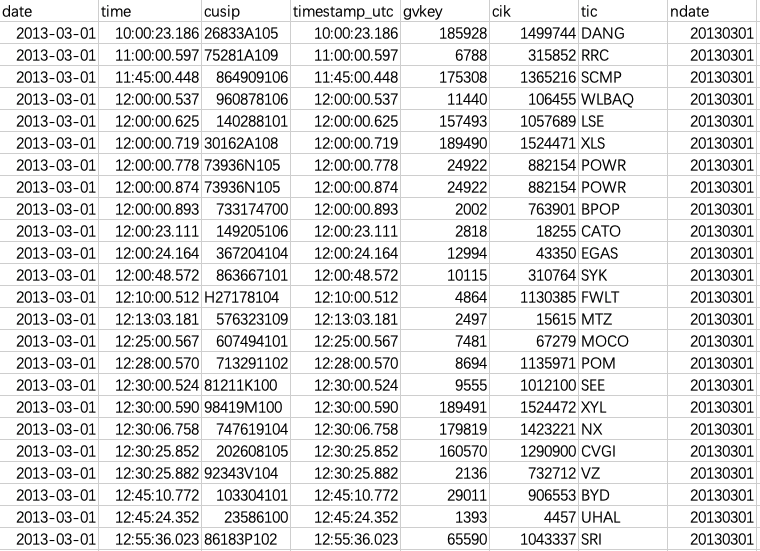
Recall a typical returned data for each tweet is as follows. suppose the root json node for a given tweet is tw
.
For each firm, there are following columns:
- Release time stamp
tw['created_at']
- Tweet ID
tw['id']
- Tweet text
tw['text']
- retweet_count
tw['public_metrics']['retweet_count']
- reply_count
tw['public_metrics']['reply_count']
- like_count
tw['public_metrics']['like_count']
- Author ID
tw['author_id']
- Language of Tweet
tw['lang']
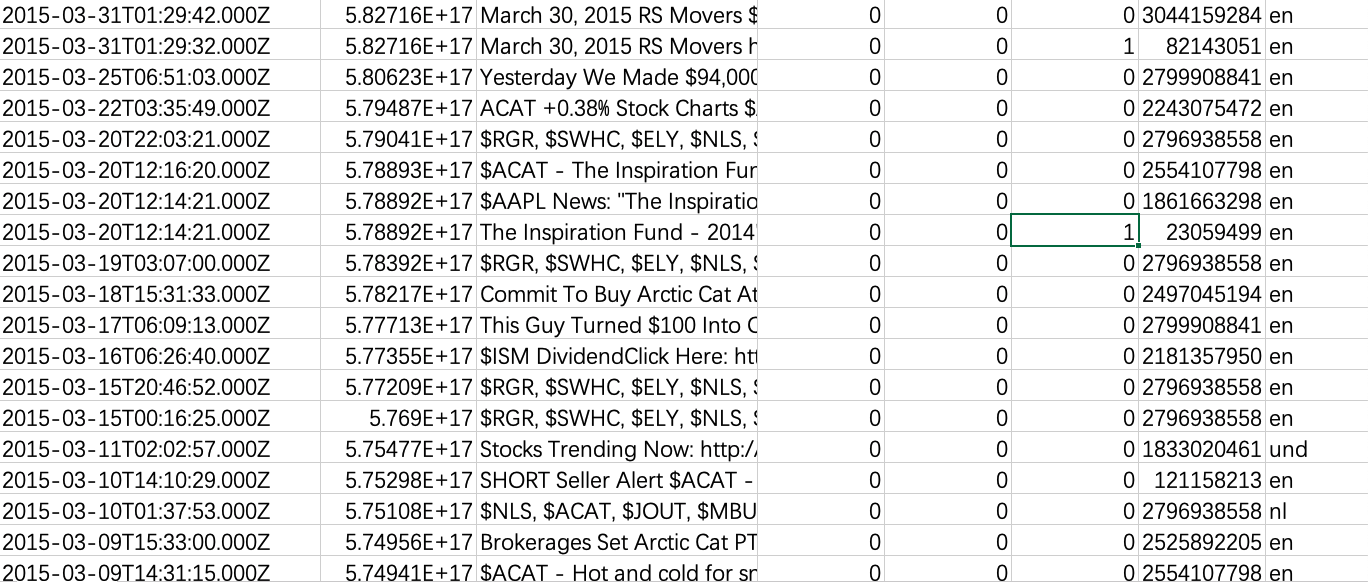
Codes
|
|
Summary and Upcoming Posts
In this blogpost, I replicated the Tweets extraction process of Bartov, Faurel, and Mohanram (2018, TAR). But retreiving the tweets archive is just a very small part of the Twitter API’s functions. In the next blogpost, I will introduce how to request the detailed user account information as well as how to check the bi-direction links among multiple Twitter accounts.
References
https://github.com/twitterdev/Twitter-API-v2-sample-code